Overview
Private capital investments have grown exponentially over the past decades. Now they are expanding at a steadier pace which necessitates tools with a higher level of confidence and precision to keep optimizing returns. Most people in the private capital space know of the Takahashi-Alexander model. Developed at Yale University in 2001, its popularity stems from its simple, intuitive design.
At FRG, we were inspired to challenge this base model by creating our own, with the goal of creating not only a more accurate model but expanding it to incorporate real-world business factors. The result? A much more informed model based on decades of data spanning thousands of funds that can be stress tested against macroeconomic scenarios and other potential future events.
The table below summarizes the main differences between the FRG model and the Takahashi-Alexander model (also called the Yale model). This blog will serve to break down each of these points and reveal how all of these together provide a more realistic model.
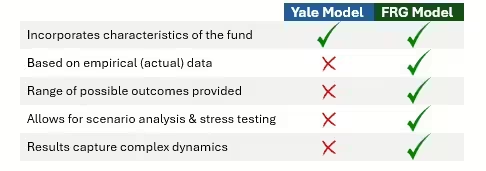
Performance Differences
When tested against empirical (actual) data, the Takahashi-Alexander model captured the general exponential trend, but the FRG model was able to make predictions much closer to the realized values. Unlike the Takahashi-Alexander model, the FRG model is not fitted to a particular line or curve, offering more sensitivity to factors that could impact future cash flows. The graph below demonstrates these performance differences by showing the comparison between the probability of call for Yale’s model (the base model) vs FRG’s model overlayed with the realized values.
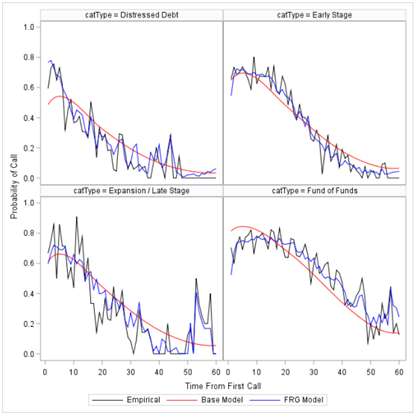
Expanding the Base Formula
So, how were we able to develop a model with such significant improvement over the industry standard? FRG partnered with Preqin to test the Takahashi-Alexander model against their large database consisting of thousands of private capital funds. Our team found that while there is some relationship between the probability and amount of each cash flow occurring, separating those into distinct models allows for higher predictive power. These models are the probability of call, the magnitude of call, the probability of distribution, and the magnitude of distribution. Each of these models has their own combination of dependent variables found to be the most informative. Aggregated together, these create the popular J Curve.
The Takahashi-Alexander model is based on what you see below— expected cash flow for a given quarter is expected contributions less expected distributions. Their model incorporates time and attributes of the fund to estimate each capital call and distribution for a given period, however these are based on assumptions or estimations and not empirical data.


To allow for greater insight from our resulting model, the Monte Carlo method was applied so that thousands of iterations of the forecast would run, and full distribution statistics could be provided. That way, rather than offering a single output, a range of possible outcomes are produced via distribution statistics.
Incorporating macroeconomic scenarios
Portfolio stress testing is FRG’s bread and butter. So, naturally, we saw an opportunity to apply scenario and stress testing analysis to private capital forecasting. This was accomplished in part by incorporating macroeconomic scenarios to help inform the model, which can be adjusted to perform stress testing. This is a marked difference from the Yale model, which only incorporates internal factors of the fund.
FRG provides three macroeconomic scenarios out of the box— baseline, mild recession, and severe recession. These are based on macroeconomic data going back several decades and cycles, updated quarterly under the advisement of an economist. There are also additional scenarios available for purchase, or users can apply their custom scenarios.
Conclusion
Since 2001, the private capital space has exploded in popularity and scope, requiring a much-needed update to the way cash flows are forecasted. In response, FRG has provided a modern approach to vastly improve the industry standard Takahashi-Alexander model. Given that a range of distribution statistics are provided, and scenario analysis and stress testing can be performed, the FRG model also has the advantage of additional insight that the Takahashi-Alexander model was simply not built for. In that same spirit of continued innovation, FRG regularly tests its models against the latest empirical data to ensure that it is always the best it can be.
For more information…
Interested in learning more? Request a demo.
Our Resources page has further reading:
- Optimal Pacing for Private Capital Portfolios—to learn about FRG’s pacing optimization tool
- Macroeconomic Effects on the Modeling of Private Capital Cash Flows—a white paper that discusses efforts undertaken to address investors’ need for Private Capital cash flow information, and some of the methodology applied to achieve the results.
Jenny Harrod is a business analytics consultant at FRG. Since joining FRG, she has become involved with FRG’s Private Capital Forecasting solution, assisting with implementation for clients and providing ad-hoc analysis. She also performs exploratory data analysis, builds machine learning algorithms, and writes and maintains technical documentation.