One of my favorite “Steve” memes is:
Steve joined a gym in January. He’s had disappointing results. Today, he has finally decided to head down there himself to find out what’s going on. -In Otter News
I am not saying that banks have treated AI like Steve’s gym membership, but a couple of recent reports nod discreetly in that direction.
In all of our exhilaration and hype about AI being the next magic wand that can fix everything from stale bread to the math problem of whether general elephants have at most du Val singularities, we seem to gloss over the fact that there are prerequisites and resources needed for it to do so.
While banking has been called out as the industry that potentially could benefit the most from AI, it is also an industry known for its technical debt, which is just a fancy word for the ever-increasing cost of servicing and building on top of outdated legacy systems and old code.
As is the case with most debt, if you do not pay it off, you end up pushing it in front of you where it obscures your view.
A lot of these legacy systems are not scaled for AI or its little brother, Machine Learning (ML). Many of them are converted from (or are still) on-prem to cloud, with highly siloed data structures and manual- to poor-connectivity between these systems and the rest of the world.
Not only is it difficult (and costly) to train AI models on such a patchwork, but it also poses security and performance blind spots simply because there is no precedence for them being online and available 24/7.
A special report from the Financial Times talks about numerous financially and reputationally damaging outages at both Barclays (33), HSBC, and Santander (both 32) in the past two years. And that is not mentioning the failures that can snowball from one small input error in an old-school backup system to accidentally crediting a client account with $81TN—a Citigroup oopsie from last year.
But it is one thing is to stop doing it wrong. It is another challenge to start doing it right. With data being vast, fragmented, and disorganized, it takes a hybrid skillset of understanding both bank business and data science to get the most out of AI, and such profiles are hard to come by.
Last week, The Banker published an article about how banks are frantically searching for data scientists who know finance and risk, and how AI can automate them out of a job (as my boss says while he points to himself).
It is a small consolation, as WSJ wrote in a recent article, that it is perfectly normal for industries to experience a J-curve in return and productivity when adopting a new technology such as AI. It takes time, (human) expertise, and practice to reach full scale.
As with the gym membership, you have to show up in person to get in better shape.
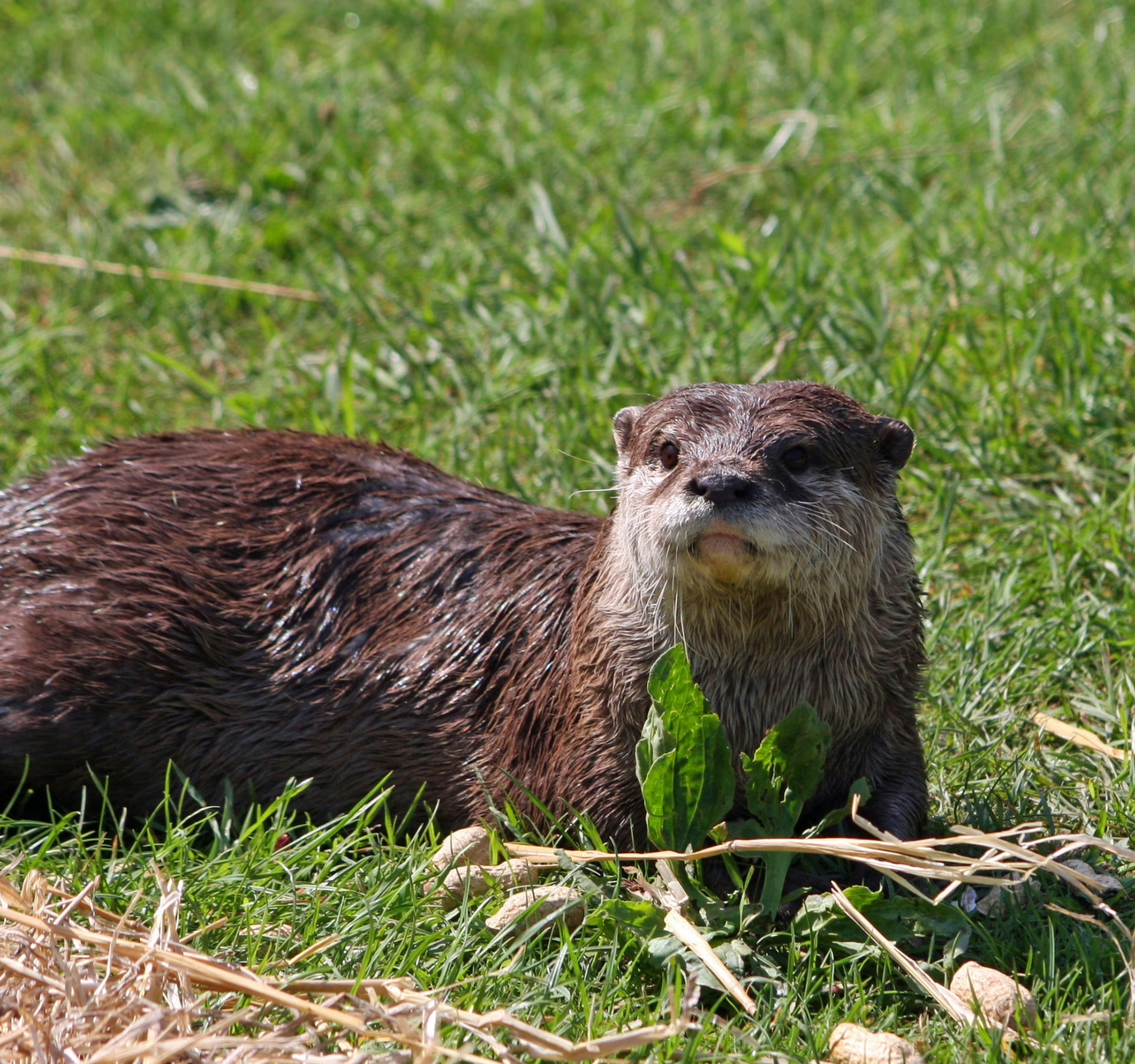
Regitze Ladekarl, FRM, is FRG’s Director of Company Intelligence. She has 25-plus years of experience where finance meets technology.
This article is part of the FRG Risk Report, published weekly on the FRG blog. To read other entries of the Risk Report, visit frgrisk.com/category/risk-report/.